Catalogue Search | MBRL
نتائج البحث
MBRLSearchResults
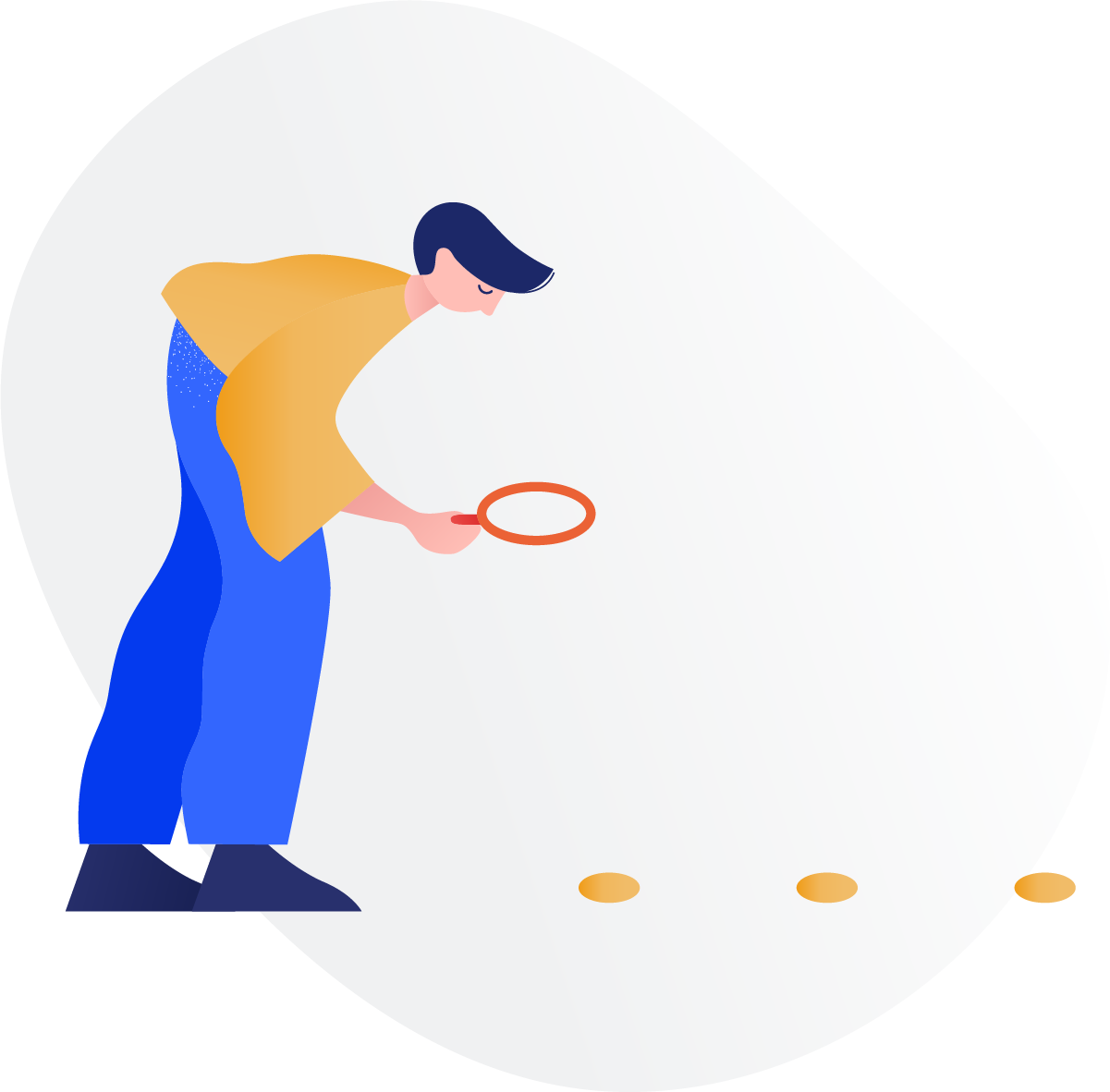
وجه الفتاة! هناك خطأ ما.
أثناء محاولة إضافة العنوان إلى الرف ، حدث خطأ ما :( يرجى إعادة المحاولة لاحقًا!
-
الضبطالضبط
-
مُحَكَّمةمُحَكَّمة
-
مستوى القراءةمستوى القراءة
-
نوع المحتوىنوع المحتوى
-
السنةمن:-إلى:
-
المزيد من المرشحاتالمزيد من المرشحاتنوع العنصرلديه النص الكاملالموضوعالناشرالمصدرالمُهدياللغةمكان النشرالمؤلفينالموقع
منجز
مرشحات
إعادة تعيين
13
نتائج ل
"Basu, Meheli"
صنف حسب:
Consuming in a crisis: pandemic consumption across consumer segments and implications for brands
بواسطة
Basu, Meheli
,
Swaminathan, Vanitha
في
Anniversaries
,
Consumer attitudes
,
Consumer behavior
2023
Purpose
This paper aims to understand how the Covid-19 pandemic has changed consumers’ perceptions of outdoor consumption categories, such as retail shopping, eating out, public events and travel and how these perceptions may impact businesses in these domains in the long term. Further, this research aims to understand demographic effects on outdoor consumption inhibition during the current pandemic and discuss how businesses can use these insights to rebrand their offerings and evolve after the pandemic.
Design/methodology/approach
Data collected by CivicScience, a survey-based consumer intelligence research platform, during April–July 2020 forms the basis of the preliminary analysis, where the chi-square test has been used to examine significant differences in consumer attitudes between different age groups, income groups and genders. Further, a social media analysis of conversations around outdoor consumption activities is undertaken to understand the rationale behind these demographics-based attitude differences.
Findings
Results lend varying degrees of support to the hypothesized consumer attitudes toward outdoor consumption activities during the Covid-19 pandemic. As the pandemic wore on, older (vs younger), female (vs male) consumers and lower (vs higher) income-group consumers had reportedly higher inhibition toward different outdoor activities. Older individuals were significantly less likely to shop, dine and attend public events than younger individuals. Lower-income consumers were significantly less likely to dine and travel than higher-income consumer consumers. Female consumers were significantly less likely to shop and travel than male consumers. Social media scan of conversations suggests that differences in perceived health and financial risks may have resulted in demographics-based differences in outdoor consumption activities.
Research limitations/implications
This study contributes to the literature by understanding demographic differences in consumer participation in outdoor activities. One limitation is that due to the time-sensitive nature of the pandemic research, further studies could not be conducted to understand the implications of other variables, beyond demographics that influence consumer behavior during a crisis. A future research direction is to understand how other psychological variables or traits, influence health and financial risk-taking behavior during a similar crisis.
Originality/value
The principal contribution of the present research is that it tests the risk-taking theory in the context of outdoor consumption during the Covid-19 pandemic. The present research has implications for businesses as they continue to evolve during and post Covid-19.
Journal Article
A gated recurrent unit approach to bitcoin price prediction
بواسطة
Dutta, Aniruddha
,
Kumar, Saket
,
Basu, Meheli
في
Accuracy
,
Algorithms
,
Artificial intelligence
2020
In today's era of big data, deep learning and artificial intelligence have formed the backbone for cryptocurrency portfolio optimization. Researchers have investigated various state of the art machine learning models to predict Bitcoin price and volatility. Machine learning models like recurrent neural network (RNN) and long short-term memory (LSTM) have been shown to perform better than traditional time series models in cryptocurrency price prediction. However, very few studies have applied sequence models with robust feature engineering to predict future pricing. In this study, we investigate a framework with a set of advanced machine learning forecasting methods with a fixed set of exogenous and endogenous factors to predict daily Bitcoin prices. We study and compare different approaches using the root mean squared error (RMSE). Experimental results show that the gated recurring unit (GRU) model with recurrent dropout performs better than popular existing models. We also show that simple trading strategies, when implemented with our proposed GRU model and with proper learning, can lead to financial gain.
Journal Article
Empirical Studies in Consumer Shopping Journey: two Essays Examining Consumer Information Search and Purchase
2020
This dissertation is directed toward understanding shoppers’ search and purchase processes in both online and offline domains. The first essay in my dissertation examines shoppers’ online omni-channel path to purchase. The second essay analyzes shoppers’ search and purchase in an offline grocery store. In essay 1, I study search and purchase behavior in the digital domain. I examine conversion rates on mobile versus desktop for deadline driven purchases (viz., event tickets) to see how the consumer’s choice of device in the digital path to purchase, influences conversion rates. The path from start to finish is mapped by using browsing and transaction data provided by StubHub and by applying econometric modeling, I study the impact of device switching vs single device use and interaction of device choice with time to event to determine purchase outcomes. The results show that the conversion rate for a PC-only web path is significantly higher than a mobile only path. Purchase likelihood significantly decreases with device switching and is higher for PC-to-mobile switching than for the reverse. In studying the interaction between time-to-event from initial search and device used, initial search on a mobile device results in a lower probability of purchase for distant consumption. Experimental results show that search inconvenience associated with mobile use outweighs purchase risk concerns to impede purchase on mobile. In essay 2, I study shoppers’ sensitivity to nutritional promotions and consequent purchase in the offline domain. This research examines how shopper reaction to nutritional information in retailer promotions impact sales. By analyzing frequent shopper data for 40,000 shoppers during a twenty-seven month period, provided by a regional supermarket chain, this research examines whether sensitivity toward price promotions is affected by heightening the salience of nutritional information via featuring nutrition promotions in the grocery chain’s weekly circular. The central hypothesis is that product level sales is improved by featuring nutrition promotions. Importantly, this research investigates the spill-over effects of nutrition promotions on sales of other products in the same category and the sales of products in a different category. The moderating effects of shopper characteristics (nutrition consciousness and share-of-wallet) and category characteristics (higher vs lower nutrition category) on the effect of nutrition promotion on sales are further studied. Simply adding nutritional information in the price promotion circulars is found to lead to additional increase in weekly product level and category sales. Interestingly, shoppers with higher nutrition consciousness and lower spending at the retailer store react more positively to nutrition promotions. Results also indicate that nutritional information display improves sales more, when featured in lower nutrition categories.
Dissertation
Empirical Studies in Consumer Shopping Journey: Two Essays Examining Consumer Information Search and Purchase
2020
This dissertation is directed toward understanding shoppers’ search and purchase processes in both online and offline domains. The first essay in my dissertation examines shoppers’ online omni-channel path to purchase. The second essay analyzes shoppers’ search and purchase in an offline grocery store. In essay 1, I study search and purchase behavior in the digital domain. I examine conversion rates on mobile versus desktop for deadline driven purchases (viz., event tickets) to see how the consumer’s choice of device in the digital path to purchase, influences conversion rates. The path from start to finish is mapped by using browsing and transaction data provided by StubHub and by applying econometric modeling, I study the impact of device switching vs single device use and interaction of device choice with time to event to determine purchase outcomes. The results show that the conversion rate for a PC-only web path is significantly higher than a mobile only path. Purchase likelihood significantly decreases with device switching and is higher for PC-to-mobile switching than for the reverse. In studying the interaction between time-to-event from initial search and device used, initial search on a mobile device results in a lower probability of purchase for distant consumption. Experimental results show that search inconvenience associated with mobile use outweighs purchase risk concerns to impede purchase on mobile. In essay 2, I study shoppers’ sensitivity to nutritional promotions and consequent purchase in the offline domain. This research examines how shopper reaction to nutritional information in retailer promotions impact sales. By analyzing frequent shopper data for 40,000 shoppers during a twenty-seven month period, provided by a regional supermarket chain, this research examines whether sensitivity toward price promotions is affected by heightening the salience of nutritional information via featuring nutrition promotions in the grocery chain’s weekly circular. The central hypothesis is that product level sales is improved by featuring nutrition promotions. Importantly, this research investigates the spill-over effects of nutrition promotions on sales of other products in the same category and the sales of products in a different category. The moderating effects of shopper characteristics (nutrition consciousness and share-of-wallet) and category characteristics (higher vs lower nutrition category) on the effect of nutrition promotion on sales are further studied. Simply adding nutritional information in the price promotion circulars is found to lead to additional increase in weekly product level and category sales. Interestingly, shoppers with higher nutrition consciousness and lower spending at the retailer store react more positively to nutrition promotions. Results also indicate that nutritional information display improves sales more, when featured in lower nutrition categories.
Dissertation
Optimal dispatch in smart power grids with partially known deviation
2015
Power grid is an interconnected system of supplying electricity from the supplier to the consumer, consisting of electricity generating plant, high voltage transmission lines- to carry electricity from the generating plant to the load center, and distribution lines- to carry electricity from load centers to individual consumers. A lot of research is being pursued to develop technologies for improving the next generation of power grid called the Smart Power Grid. The Smart Power Grid will have sophisticated communication infrastructure to improve the efficiency of electricity generation using renewable energy sources like the sun, water, etc and also to inform consumers of their electricity usage pattern. Also, the electricity market is now divided into three sections- generation, transmission and distribution. Private companies are competing with each other to provide electricity at the most competitive market price. We have developed two algorithms to help generating companies achieve their goal of meeting the hourly electricity need of the consumers and to do so at a minimum total cost.
Dissertation
A Gated Recurrent Unit Approach to Bitcoin Price Prediction
بواسطة
Dutta, Aniruddha
,
Kumar, Saket
,
Basu, Meheli
في
Artificial intelligence
,
Digital currencies
,
Machine learning
2019
In today's era of big data, deep learning and artificial intelligence have formed the backbone for cryptocurrency portfolio optimization. Researchers have investigated various state of the art machine learning models to predict Bitcoin price and volatility. Machine learning models like recurrent neural network (RNN) and long short-term memory (LSTM) have been shown to perform better than traditional time series models in cryptocurrency price prediction. However, very few studies have applied sequence models with robust feature engineering to predict future pricing. in this study, we investigate a framework with a set of advanced machine learning methods with a fixed set of exogenous and endogenous factors to predict daily Bitcoin prices. We study and compare different approaches using the root mean squared error (RMSE). Experimental results show that gated recurring unit (GRU) model with recurrent dropout performs better better than popular existing models. We also show that simple trading strategies, when implemented with our proposed GRU model and with proper learning, can lead to financial gain.
Paper
An Efficient Convolutional Neural Network for Coronary Heart Disease Prediction
بواسطة
Batabyal, Tamal
,
Dutta, Aniruddha
,
Acton, Scott T
في
Accuracy
,
Artificial neural networks
,
Cardiovascular disease
2020
This study proposes an efficient neural network with convolutional layers to classify significantly class-imbalanced clinical data. The data are curated from the National Health and Nutritional Examination Survey (NHANES) with the goal of predicting the occurrence of Coronary Heart Disease (CHD). While the majority of the existing machine learning models that have been used on this class of data are vulnerable to class imbalance even after the adjustment of class-specific weights, our simple two-layer CNN exhibits resilience to the imbalance with fair harmony in class-specific performance. In order to obtain significant improvement in classification accuracy under supervised learning settings, it is a common practice to train a neural network architecture with a massive data and thereafter, test the resulting network on a comparatively smaller amount of data. However, given a highly imbalanced dataset, it is often challenging to achieve a high class 1 (true CHD prediction rate) accuracy as the testing data size increases. We adopt a two-step approach: first, we employ least absolute shrinkage and selection operator (LASSO) based feature weight assessment followed by majority-voting based identification of important features. Next, the important features are homogenized by using a fully connected layer, a crucial step before passing the output of the layer to successive convolutional stages. We also propose a training routine per epoch, akin to a simulated annealing process, to boost the classification accuracy. Despite a 35:1 (Non-CHD:CHD) ratio in the NHANES dataset, the investigation confirms that our proposed CNN architecture has the classification power of 77% to correctly classify the presence of CHD and 81.8% the absence of CHD cases on a testing data, which is 85.70% of the total dataset. ( (<1920 characters)Please check the paper for full abstract)
Paper
A Gated Recurrent Unit Approach to Bitcoin Price Prediction
بواسطة
Kumar, Saket
,
Dutta, Aniruddha
,
Basu, Meheli
في
Artificial intelligence
,
Digital currencies
,
Machine learning
2019
In today's era of big data, deep learning and artificial intelligence have formed the backbone for cryptocurrency portfolio optimization. Researchers have investigated various state of the art machine learning models to predict Bitcoin price and volatility. Machine learning models like recurrent neural network (RNN) and long short-term memory (LSTM) have been shown to perform better than traditional time series models in cryptocurrency price prediction. However, very few studies have applied sequence models with robust feature engineering to predict future pricing. in this study, we investigate a framework with a set of advanced machine learning methods with a fixed set of exogenous and endogenous factors to predict daily Bitcoin prices. We study and compare different approaches using the root mean squared error (RMSE). Experimental results show that gated recurring unit (GRU) model with recurrent dropout performs better better than popular existing models. We also show that simple trading strategies, when implemented with our proposed GRU model and with proper learning, can lead to financial gain.
Paper
Multidisciplinary management of ovarian germ cell tumours-a single institutional study from India
2021
Ovarian germ cell tumours constitute a heterogeneous group of neoplasm with malignant potential being seen in 5% of cases. There is limited data on treatment outcomes of patients with malignant ovarian germ cell tumours (MOGCT). Here, we present our hospital audit of patients with MOGCT.
This is a retrospective data review of patients with MOGCT treated between May 2011 and December 2019. Patients were treated with staging laparotomy and adjuvant chemotherapy, wherever applicable. Surveillance was allowed for those at low risk for recurrence. Clinicopathologic features and treatment details were recorded, and survival analysis was performed.
Sixty-five patients with a median age of 25 years (range: 11-52 years) were treated during the study period. The most common histology was immature teratoma in 35.3% of cases. International Federation of Gynecology and Obstetrics stage IC was the most common stage of presentation (47%). Surveillance was advised for 12.3% of cases. Systemic therapy was given in 51 (78%) patients. At a median follow-up of 46 months (range: 1-109 months), the median progression-free survival (PFS) was not reached. Five-year PFS was 79.3% (95% CI: 65.8-88). The most common toxicity was febrile neutropenia (22%) among those who received systemic therapy.
Immature teratoma was the most common histology in our series. The majority presented in the early stage. MOGCT is a highly curable disease with surgery and systemic therapy.
Journal Article